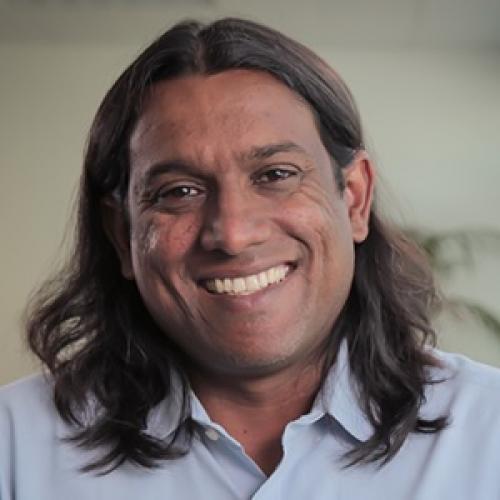
Zaid Kahn
Zaid is currently GM in Cloud Hardware Infrastructure Engineering where he leads a team focusing on advanced architecture and engineering efforts for AI. He is passionate about building balanced teams of artists and soldiers that solve incredibly difficult problems at scale.
Prior to Microsoft Zaid was head of infrastructure engineering at LinkedIn responsible for all aspects of engineering for Datacenters, Compute, Networking, Storage and Hardware. He also lead several software development teams spanning from BMC, network operating systems, server and network fleet automation to SDN efforts inside the datacenter and global backbone including edge. He introduced the concept of disaggregation inside LinkedIn and pioneered JDM with multiple vendors through key initiatives like OpenSwitch, Open19 essentially controlling destiny for hardware development at LinkedIn. During his 9 year tenure at LinkedIn his team scaled network and systems 150X, members from 50M to 675M, and hiring someone every 7 seconds on the LinkedIn Platform.
Prior to LinkedIn Zaid was Network Architect at WebEx responsible for building the MediaTone network and later I built a startup that built a pattern recognition security chip using NPU/FPGA. Zaid holds several patents in networking and SDN and is also a recognized industry leader. He previously served as a board member of the Open19 Foundation and San Francisco chapter of Internet Society. Currently he serves on DE-CIX and Pensando advisory boards.