Disaggregated memory is a promising approach that addresses the limitations of traditional memory architectures by enabling memory to be decoupled from compute nodes and shared across a data center. Cloud platforms have deployed such systems to improve overall system memory utilization, but performance can vary across workloads. High-performance computing (HPC) is crucial in scientific and engineering applications, where HPC machines also face the issue of underutilized memory. As a result, improving system memory utilization while understanding workload performance is essential for HPC operators. Therefore, learning the potential of a disaggregated memory system before deployment is a critical step. This paper proposes a methodology for exploring the design space of a disaggregated memory system. It incorporates key metrics that affect performance on disaggregated memory systems: memory capacity, local and remote memory access ratio, injection bandwidth, and bisection bandwidth, providing an intuitive approach to guide machine configurations based on technology trends and workload characteristics. We apply our methodology to analyze thirteen diverse workloads, including AI training, data analysis, genomics, protein, fusion, atomic nuclei, and traditional HPC bookends. Our methodology demonstrates the ability to comprehend the potential and pitfalls of a disaggregated memory system and provides motivation for machine configurations. Our results show that eleven of our thirteen applications can leverage injection bandwidth disaggregated memory without affecting performance, while one pays a rack bisection bandwidth penalty and two pay the system-wide bisection bandwidth penalty. In addition, we also show that intra-rack memory disaggregation would meet the application's memory requirement and provide enough remote memory bandwidth.
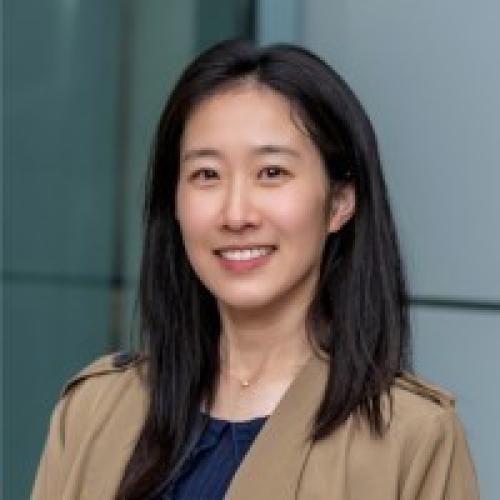
Nan Ding
Nan Ding is a Research Scientist in the Performance and Algorithms group of the Computer Science Department at Lawrence Berkeley National Laboratory. Her research interests include high-performance computing, performance modeling and performance optimization. Nan received her Ph.D. in computer science from Tsinghua University, Beijing, China in 2018.