Memory Optimizations for Machine Learning
As Machine Learning continues to forge its way into diverse industries and applications, optimizing computational resources, particularly memory, has become a critical aspect of effective model deployment. This session, "Memory Optimizations for Machine Learning," aims to offer an exhaustive look into the specific memory requirements in Machine Learning tasks and the cutting-edge strategies to minimize memory consumption efficiently.
We'll begin by demystifying the memory footprint of typical Machine Learning data structures and algorithms, elucidating the nuances of memory allocation and deallocation during model training phases. The talk will then focus on memory-saving techniques such as data quantization, model pruning, and efficient mini-batch selection. These techniques offer the advantage of conserving memory resources without significant degradation in model performance.
Additional insights into how memory usage can be optimized across various hardware setups, from CPUs and GPUs to custom ML accelerators, will also be presented.
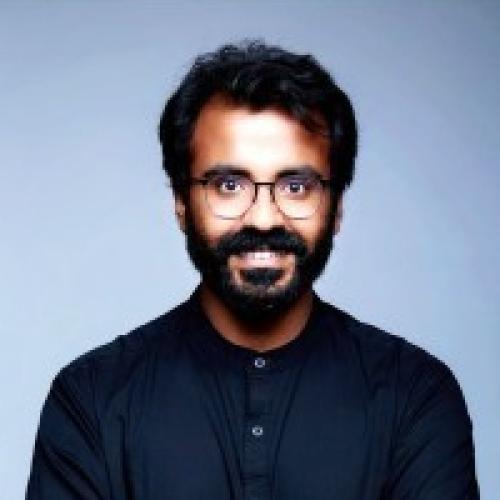
Tejas Chopra
Tejas Chopra is a Sr. Engineer at Netflix working on Machine Learning Platform for Netflix Studios and a Founder at GoEB1 which is the world’s first and only thought leadership platform for immigrants.Tejas is a recipient of the prestigious EB1A (Einstein) visa in US. Tejas is a Tech 40 under 40 Award winner, a TEDx speaker, a Senior IEEE Member, an ACM member, and has spoken at conferences and panels on Cloud Computing, Blockchain, Software Development and Engineering Leadership.Tejas has been awarded the ‘International Achievers Award, 2023’ by the Indian Achievers’ Forum. He is an Adjunct Professor for Software Development at University of Advancing Technology, Arizona, an Angel investor and a Startup Advisor to startups like Nillion. He is also a member of the Advisory Board for Flash Memory Summit.Tejas’ experience has been in companies like Box, Apple, Samsung, Cadence, and Datrium. Tejas holds a Masters Degree in ECE from Carnegie Mellon University, Pittsburgh.